🚀 Reaching new heights: EvolutionIQ and CCC Intelligent Solutions join forces to redefine the future of claims! 🎉
Understanding the Timelines of Insurance Claims to Predict Future Outcomes Using AI
How can we expect examiners to make consistent, fair and accurate decisions when their experience is limited to a small subset of the claims they’ve personally …

How can we expect examiners to make consistent, fair and accurate decisions when their experience is limited to a small subset of the claims they’ve personally reviewed?
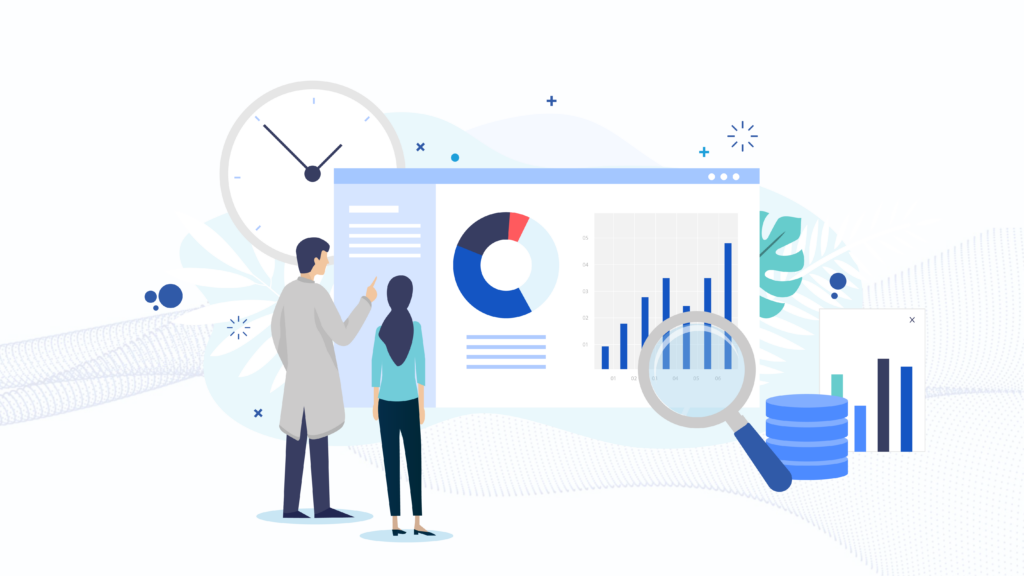
Tech-forward industries are benefiting from human-machine partnerships, helping drive better, faster and more accurate decision making by surfacing all the key lessons of the past to individual professionals tasked with making final determinations. At EvolutionIQ, we’re bringing that future to insurance, by partnering with adjusters, managers and claims executives to drive the most critical lessons learned over the entire organizational experience to the precise adjuster or examiner working the most relevant claim.
In order to deliver the key insights at precisely the right moment, our AI learning algorithms mimic how examiners discern best practices and how the claims process evolved over years or even decades during their reviews. For example, examiners learn to recognize specific language and patterns in nurse and doctor notes and how claimants interact with the insurance carrier. This insight and experience allows examiners to know what to do when they get to review a new claim. Hence if AI can pick up on similar patterns and even discover new ones, it can support examiners in their work.
Insurance claims data pose unique challenges to AI algorithms for two main reasons:
- the majority of the data is in an unstructured format, including examiner notes and correspondence, and
- the importance of understanding data in a time series of months, if not years, that a claim might remain open.
For example, in the illustration below the claimant got an initial diagnosis. Then the examiner left a voicemail for the claimant and subsequently ordered a comprehensive vocational review. The sequence continued when the examiner ordered a recent medical review that provided updated diagnosis and prognosis. Based on this information, the examiner has to make a decision on whether the presented information supports ongoing disability payments.
Learning about claims: Human version

For illustration purposes, let’s assume the examiner concludes that the claimant can return to work based on the vocational review and the latest medical update, so sends out a letter to close the claim. Thus, the examiner decides, based on experience, that the more recent doctor’s note supercedes the previous diagnosis.The fact that the examiner left a voicemail was not informative in determining the validity of an ongoing disability.
Once examiners look at all the historical information of a claim, they summarize it in a way that is useful to predict the future and inform decisions — a learning algorithm. They are not merely repeating all the historical information, but reducing it to the critical facts that had an impact on how they want to handle the claim.
Learning about claims: Machine version
Artificial Intelligence can learn the same information, but not from the same lesson plan.
Time series or sequence machine learning models behave similarly to their human counterparts, but capitalize on significantly more data points. Essentially, they take the entire array of past and present events and use that to more accurately predict the future. In effect, AI determines which patterns are useful and which are not, then relies on the greater predictive value of the more useful data to make more accurate forecasts.
More formally, EvolutionIQ aims to learn about the future ability to RTW given past and present claims data:

An ML algorithm tries to learn this as a function which maps claims data to a probability score) for each claim at any given point in time.
f(claims data from present and past) → score
In the example above, examiners can rely on their experience to know which subset of information was important to predict the future — voc review, latest medical review — and what subset was safe to ignore — voicemail, outdated diagnosis. This “experience” is exactly what historical claims data can teach ML algorithms. At EvolutionIQ we use sequence models, such as recurrent neural networks or long short-term memory models to learn important dynamic patterns that can achieve state-of-the-art forecasting performance.
Live Claim example explained using AI
On the flipside, EvolutionIQ also invests heavily into explainable AI in order to avoid the pitfalls of so-called “black-box” solutions. We want our clients to understand the decision making that goes into these algorithms. As a concrete example, consider the claim below, which was opened in January 2011 and was eventually closed in March 2018. The y-axis represents our AI’s prediction about the likelihood of a claim to be closed and denied in the near future. The time series chart shows the evolution of how this forecast of the claim’s future changes as time goes on. For the first two years after approval there is no evidence to support claim closure. Starting early 2013, though, evidence to support closure starts to slightly increase, followed by two big increases in Feb 2014 and March 2015. Our explainable AI technology tells us that the first jump can be attributed to a claim status change by insurance, the second to an update in ICD medical codes applied based on examiner notes. This also illustrates the critical feedback loop between users and AI technology as they learn from each other. Gradual increases after March 2015 are primarily driven by the time since the claim status has changed (back in Feb 2014) as well as medical updates in examiner notes.
Crucially, EvolutionIQ’s systematic Artificial Approach to understanding the claim would have flagged it for review & closure in 2016, whereas it was not closed for almost 3 more years.
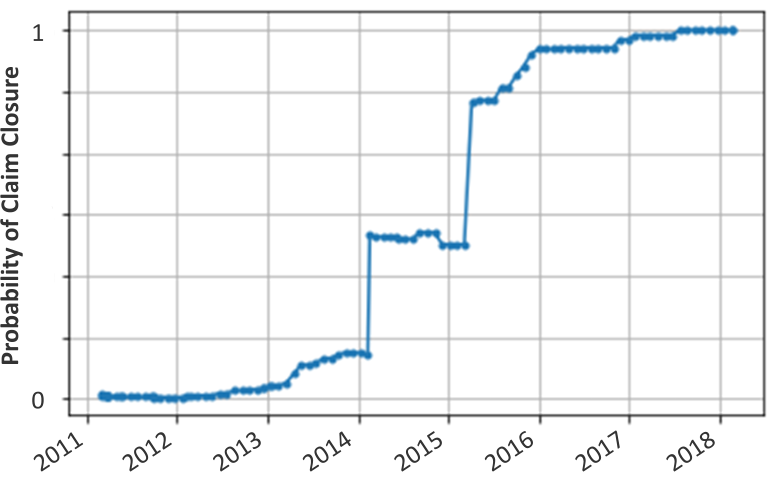
Ultimately this approach to analyzing claims provides a more nuanced understanding of severity and can more accurately map actions to outcomes. Rule- or feature-based systems often rely on predefined correlations between an activity or diagnosis and severity. As we have seen in the example above, though, the timing and sequence of events in a claim’s life cycle ultimately impact the claim differently. By tying the pattern of activity on a claim to the ultimate outcomes we can give claims handlers and adjusters a great deal of early insight to prioritize and streamline their workflow.
If you’d like to know more about how this technology relates to your company’s unique position, request a EvolutionIQ demo.